Artificial bee colony algorithm
Post-publication activity
Curator: Dervis Karaboga
Contributors:
0.40 -
James Meiss
0.20 -
Benjamin Bronner
Eugene M. Izhikevich
Florian Hauser
The Artificial Bee Colony (ABC) algorithm is a swarm based meta-heuristic algorithm that was introduced by Karaboga in 2005 () for optimizing numerical problems. It was inspired by the intelligent foraging behavior of honey bees. The algorithm is specifically based on the model proposed by for the foraging behaviour of honey bee colonies. The model consists of three essential components: employed and unemployed foraging bees, and food sources. The first two components, employed and unemployed foraging bees, search for rich food sources, which is the third component, close to their hive. The model also defines two leading modes of behaviour which are necessary for self-organizing and collective intelligence: recruitment of foragers to rich food sources resulting in positive feedback and abandonment of poor sources by foragers causing negative feedback.
In ABC, a colony of artificial forager bees (agents) search for rich artificial food sources (good solutions for a given problem). To apply ABC, the considered optimization problem is first converted to the problem of finding the best parameter vector which minimizes an objective function. Then, the artificial bees randomly discover a population of initial solution vectors and then iteratively improve them by employing the strategies: moving towards better solutions by means of a neighbour search mechanism while abandoning poor solutions.
Contents
Global Optimization ProblemA global optimization problem can be defined as finding the parameter vector \(\vec x\) that minimizes an objective function \(f (\vec x )\ :\)
\[\tag{1} {\rm minimize~} f(\vec x),~~\vec x = (x_1 , x_2, \ldots ,x_i, \ldots,x_{n-1}, x_n ) \in \mathbb{R}^n \]
which is constrained by the following inequalities and/or equalities:
\[\tag{2} {\rm ~~~~~~~~~~~~~~~~~~~~~~}l_i \leq x_i\leq u_i, ~~~i=1,\ldots,n \]
\[\tag{3}
{\rm subject~to:~}~~~g_j( \vec{x} ) \leq 0,~{\rm for} ~~j=1,\ldots,p
\]
\[\tag{4}
{\rm ~~~~~~~~~~~~~~~~~~~} h_j(\vec{x})=0,~ {\rm for}~ ~j=p+1,\ldots,q
\]
\(f (\vec x )\) is defined on a search space, \(S\ ,\) which is a \(n-\)dimensional rectangle in \(\mathbb{R}^n\) (\(S \subseteq \mathbb{R}^n\)). The variable domains are limited by their lower and upper bounds ().
This problem is also known as a constrained optimization problem. If it is an unconstrained optimization problem, then both \(p=0\) and \(q=0\ .\)
The Artificial Bee Colony Meta-heuristicIn ABC, the colony of artificial bees contains three groups of bees: employed bees associated with specific food sources, onlooker bees watching the dance of employed bees within the hive to choose a food source, and scout bees searching for food sources randomly. Both onlookers and scouts are also called unemployed bees. Initially, all food source positions are discovered by scout bees. Thereafter, the nectar of food sources are exploited by employed bees and onlooker bees, and this continual exploitation will ultimately cause them to become exhausted. Then, the employed bee which was exploiting the exhausted food source becomes a scout bee in search of further food sources once again. In other words, the employed bee whose food source has been exhausted becomes a scout bee. In ABC, the position of a food source represents a possible solution to the problem and the nectar amount of a food source corresponds to the quality (fitness) of the associated solution. The number of employed bees is equal to the number of food sources (solutions) since each employed bee is associated with one and only one food source.
The general scheme of the ABC algorithm is as follows:
REPEAT
Employed Bees Phase
Onlooker Bees Phase
Scout Bees Phase
Memorize the best solution achieved so far
UNTIL(Cycle=Maximum Cycle Number or a Maximum CPU time)
Initialization Phase
All the vectors of the population of food sources, \(\vec{x_{m}} \)’s, are initialized \((m=1...SN\ ,\) \(SN:\) population size) by scout bees and control parameters are set. Since each food source, \(\vec{x_{m}}\ ,\) is a solution vector to the optimization problem, each \(\vec{x_{m}}\) vector holds \(n\) variables, (\(x_{mi}, i=1...n\)), which are to be optimized so as to minimize the objective function.
The following definition might be used for initialization purposes ():
\[\tag{5} x_{mi}=l_{i}+rand(0,1)*(u_i-l_i)\]
where \(l_i\) and \(u_i\) are the lower and upper bound of the parameter \(x_{mi}\ ,\) respectively.
Employed bees search for new food sources (\(\vec{\upsilon_{m}}\)) having more nectar within the neighbourhood of the food source (\(\vec{x_{m}}\)) in their memory. They find a neighbour food source and then evaluate its profitability (fitness). For example, they can determine a neighbour food source \(\vec{\upsilon_{m}}\) using the formula given by equation ():
\[\tag{6} \upsilon_{mi} = x_{mi} + \phi_{mi}(x_{mi} - x_{ki})\]
相关文章:
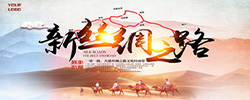
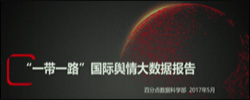